Welcome to the machine: How artificial intelligence and machine learning are revolutionizing the supply chain
November 15, 2018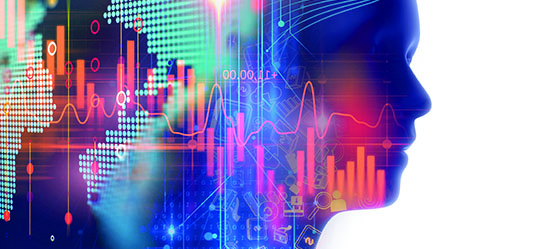
How artificial intelligence and machine learning are revolutionizing the supply chain of today— and what that means for retailers of tomorrow
BY JESSE DONALDSON
WHEN most people think of artificial intelligence (AI), the phrase conjures up images mostly pulled from pop culture:
Star Trek’s Commander Data, Schwarzenegger’s genocidal Terminator, the lovable WALL-E or the murderous robots of Westworld. Some might think of the psychedelic, nightmarish animations of Google’s Deep Dream, while others may recall Microsoft’s “Tay”—the chatbot that degenerated into racism after only a few hours of exposure to Twitter.
In the world of retail, nothing could be further from reality.
In fact, for today’s most forward-thinking retailers, AI and machine learning are already helping to transform the traditional supply chain, using complex data ecosystems to optimize replenishment, forecast demand, analyze root causes, increase efficiency, and improve the customer experience—all in a realtime environment.
“Data is the next natural resource that needs to be mined,” explains Charbel Safadi, Canadian Leader for AI and Advanced Analytics with IBM. “Every organization has that resource available to them—they have all this data— based on everything they’ve been doing just by being in business and being successful. Now they need to focus on using it properly.”
CHARBEL SAFADI, IBM
“If you think of analyzing large chunks of data, and finding what the reason for a problem is—where things went wrong, where metrics went wrong— they don’t require a human to look at all that data,” adds Alois Reitbauer, VP and Chief Technology Strategist with performance management software company Dynatrace. “Humans are very good at problem-solving on a certain level—getting the right people together, or solving problems—on a social, human-interaction level. But when it comes to working through terrabytes of data, computers are much better at doing it.”
Supply before demand?
Artificial Intelligence (AI)—the term given to machines that are able to exhibit human-like intelligence and problem-solving abilities—have actually been around in one form or another since the mid-20th Century. The first artificial neural network, made using vacuum tubes, was built at Princeton University in the early 1950s, and the first primitive AI program, Logic Theorist, was completed in 1955. However, it wasn’t until the 21st Century when the technology began to realize its true potential. Today, many tech giants have already invested a considerable amount in the area. In 2015, Google alone spent $20-$30 billion, with the bulk of that money going to AI and Machine Learning. According to a 2017 survey by the McKinsey Global Institute, retail currently falls in the “Medium Adopters” category. Of the companies surveyed, only 20 per cent have adopted AI tech at scale. But amongst those who have, the effects are already apparent: fewer returns, increased efficiency, more agile supply chains, and higher profits (according to the McKinsey study, proactive AI adopters have seen profit margins of up to 15 per cent higher than their industry average). The reasons for this are multitudinous, but chief among them is AI’s revolutionary impact on the area of demand forecasting.
“The way the retail industry has always done demand forecasting has been a very historical model,” Safadi notes. “In other words, they look backwards and say: ‘How did we do on this day, or in this segment, or in this population, or location, and what do we think the future will look like? AI and machine learning have changed the approach fundamentally. The historical data is important, certainly, but that is only one set of data points that organizations have been using to predict the future. But what we’re working on is taking additional sets of data; unstructured data—client data, weather data, IOT sensor data from a real-time perspective. And suddenly you become a lot more dynamic in your supply chain— not just when it comes to predicting the future, but in terms of how you should be operating today.”
“THE ABILITY THAT YOU HAVE, WITH A VIRTUAL OPERATIONS PERSON—YOU CAN SCALE THE ANALYSIS PART MORE OR LESS INFINITELY. YOU CAN LET THEM TAKE CARE OF MOST OF THE DATA ANALYSIS AND FOCUS YOUR EMPLOYEES ON THE THINGS THAT CAN’T BE AUTOMATED. SUDDENLY, YOUR COMPANY CAN DO WAY MORE THAN YOU COULD BEFORE.”
ALOIS REITBAUER
Dynatrace
And the benefits of this approach are already being felt by the industry’s early adopters. Using AI and machine learning—in combination with both internal and external datasets (including, as Safadi explains, such disparate items as weather forecasting, time of year, and the demographics of the neighbourhoods that each location serves)— one unnamed produce retailer has already seen an estimated 1-2 per cent increase in overall earnings. The McKinsey study posits that, with the help of AI, forecasting errors alone could be reduced by as much as 50 per cent. Lost sales due to product unavailability could be reduced by up to 65 per cent. It could mean a reduction of 5-10 per cent in transportation costs and a whopping 25-50 per cent reduction in warehousing costs. Some companies have taken this approach even further; German retailer Otto has begun using algorithms to determine how they build their entire inventory. Their AI—approximately 90 per cent accurate in forecasting customer demand—has thus far been able to reduce product returns by almost 2 million units per year.
“In terms of demand forecasting, it no longer becomes a point-in-time activity,” Safadi says. “It becomes a continuous model that improves over time and allows the forecasting to get better as more data comes into the system.”
Automatic for the people
Beyond the digital world, AI and machine learning are transforming the modern supply chain on a whole other level—within the warehouses themselves. Retail giant Amazon recently purchased Kiva, a robotics company that has allowed them to automate processes such as order picking and packing, and in so doing have reduced their “click-to-ship” cycle time from 75 minutes down to just 15 (incidentally lowering the operating costs in those facilities by 20 per cent).
Other companies have opted to use AI not to replace human workers, but to operate alongside them. Ocado in the UK has implemented a team of robots—equipped with computer vision and trained to recognize various objects—that take products from thousands of bins and deliver them to workers for packing. In fact, computer vision has become so advanced that it can differentiate between product types, and even recognize empty shelf space. Rethink Robotics, a Boston-based company, has already designed robots that can be trained by humans to perform complex tasks, and then be further refined through computer vision and machine learning. But for those worried about AI’s disruptive impact on the workforce, both Reitbauer and Safadi are quick to point out that the intention is to assist rather than to replace.
“The ability that you have, with a virtual operations person—you can scale the analysis part more or less infinitely,” Reitbauer says. “You can let them take care of most of the data analysis and focus your employees on the things that can’t be automated. Suddenly, your company can do way more than you could before.”
“Our fundamental mission is to augment human intelligence,” Safadi adds. “Everything is designed and created to extend the human capability—not to replace human intelligence. It comes back to an ethical point-of-view, and the trust and transparency in AI. From our perspective, it’s humans and machines working together that creates the value proposition. Not one over the other.”
The future is now
That said, AI has already become powerful enough that it can aid in problem-solving at virtually every step of the way. Companies like Dynatrace have spent years developing algorithms capable of doing complex Root Cause Analysis— streamlining what was once a labour-intensive, problem-solving endeavour into a process that takes a fraction of the time.
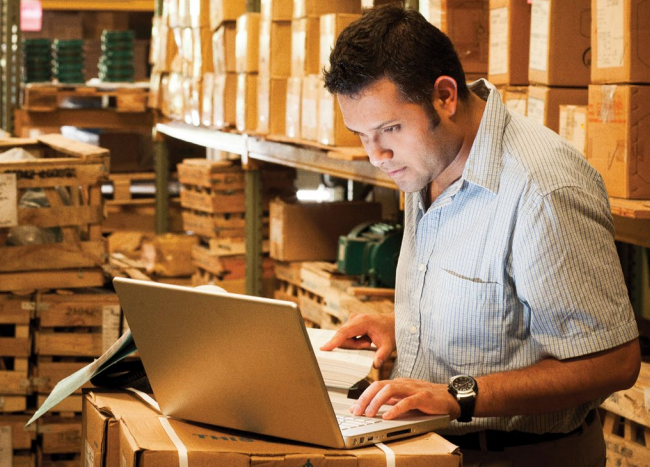
“This kind of analysis can be done in the real world, in real-time, even with billions of dependencies, in milliseconds,” Reitbauer explains. “Even a very smart human is never going to be that fast. And if you combine the output of this Root Cause Analysis with a tool that’s able to—more or less— execute an operations process automatically, then two or three minutes after a problem is detected, it can be automatically fixed without needing any human intervention. That’s a massive benefit.”
And, he adds, the applications for retailers with a digital presence are even greater—even down to the level of customer engagement and retention.
In fact, one of AI’s greatest strengths, Safadi says, is that it can allow retailers to understand their customers’ needs on a granular level. And, this knowledge means not just being able to respond to trends, but to anticipate them.
“Products shouldn’t be for the masses,” he notes. “They should be tied to the behaviours, the need, and the want of the consumer […] If you think about the history of retailers, for the most part, people have been told what products they should buy in the context of a trend. But nobody’s been able to understand why a product is emerging as a trend. To achieve that requires Big Data, so that when you’re acquiring a product in your supply chain, you’re one step ahead of the trend. We can use the weather data, along with neighbourhood data, demographic data to allow retailers to understand the behaviours of that location. If you live in a specific part of Canada, or a specific neighbourhood that’s more hip, for example, we can know that. What sort of events happen in that neighbourhood? Who lives there? And you can start to tailor your experience to that neighbourhood versus just to the masses.”
There are certainly challenges ahead. For one, Canada’s retailers tend to be relative laggards when it comes to adopting AI technology. For another, it may require some investment in infrastructure, strategic partnerships with firms like IBM and Dynatrace, integration of AI with existing datasets, as well as educating or reskilling portions of their workforce. But, for retailers who might be feeling overwhelmed by the transition into the digital ecosystem of the future, Safadi has a few solid suggestions on where and how to begin—and he’s quick to reiterate that when it comes to AI and machine learning, the future is definitely now.
“Start with the data,” he says. “This has to be top-of-mind. Companies are sitting on huge amounts of ‘gold’—this data. They need to mine that natural resource. The barrier is no longer the technology. It’s no longer the inability to find data scientists. It’s a matter of will and setting this as a priority. Because organizations who start this journey late, they’re going to significantly diminish their competitive advantage. You can’t just buy some black box full of data three years from now and say, ‘Now I want to be AI-enabled’. You need to spend the time building out these use cases. Everyone needs to start from the beginning. You can’t skip the line.”